Healthcare payers deal with a steady stream of claims every day, some accurate, many questionable. Fraudulent billing, inflated procedures, and duplicate claims continue to cost the system billions. Most detection systems are still built on rules and thresholds, which catch known patterns but often miss the subtle, evolving ones.
AI is starting to change that. Not with sweeping promises, but with small, practical wins—finding mismatches, spotting repeat behaviors, and helping teams flag issues faster. As fraud tactics become more nuanced, healthcare payers are beginning to look at AI as a way to move away from reacting to predicting.
In this blog, we’ll look at how AI is being used by healthcare payers to detect fraud more efficiently, what technologies are behind it, and where the real challenges lie.
2. Understanding Healthcare Fraud
Healthcare fraud encompasses a range of deceptive practices aimed at obtaining unauthorized benefits or payments from healthcare programs. For payers, this translates to significant financial losses and compromised trust in the system.
Common Types of Healthcare Fraud:
- Upcoding: Billing for more expensive services or procedures than those actually provided.
- Phantom Billing: Charging for services, tests, or procedures that were never performed.
- Duplicate Claims: Submitting multiple claims for the same service.
- Unbundling: Separating procedures that are typically billed together to increase reimbursement.
- Kickbacks: Offering or receiving incentives for patient referrals or services.
- Identity Theft: Using another individual’s insurance information to receive medical services.
Challenges in Detecting Fraud:
- Volume and Complexity: The sheer number of claims and the complexity of billing codes make manual detection inefficient.
- Evolving Schemes: Fraudsters continually adapt, developing new methods to exploit system vulnerabilities.
- Data Silos: Disparate data systems hinder comprehensive analysis and pattern recognition.
- Limited Resources: Many organizations lack the personnel and tools necessary for a thorough fraud investigation.
Given these challenges, traditional methods often fall short, necessitating more advanced, data-driven approaches to effectively detect and prevent fraud.
3. Traditional vs. AI-Powered Fraud Detection
How It Has Been Done (and Why It’s Not Enough)
Most payer organizations rely on rules-based systems—sets of conditions that flag claims based on pre-defined thresholds (like unusually high billing volumes or procedure mismatches). These methods are good at catching well-known schemes but struggle with:
- False positives that waste investigative time
- Fraud tactics that evolve faster than the rules
- Inflexibility when new billing codes or care models are introduced
According to findings shared in KLAS Research reports on payer IT solutions, AI adoption in fraud and payment integrity workflows is rising, especially among large health plans looking to automate claims review and reduce false positives.
Where AI Changes the Game
AI systems don’t rely solely on hardcoded rules. Instead, they learn from historical patterns to identify behavior that’s unusual even when it’s never been seen before.
For example:
- A machine learning model might flag a provider who’s billing for a rarely used procedure at 20x the regional average.
- NLP (Natural Language Processing) could parse unstructured notes in claims or attachments to detect inconsistencies in diagnoses and procedures.
- Generative AI tools are being piloted to simulate potential fraud scenarios and test a system’s ability to catch them.
Unlike traditional systems, AI tools adapt over time, getting better with more data and feedback from investigators.
4. Key AI Technologies in Healthcare Fraud Detection
AI has become an indispensable tool in identifying and preventing fraud within the healthcare sector. Several AI technologies contribute to this effort:
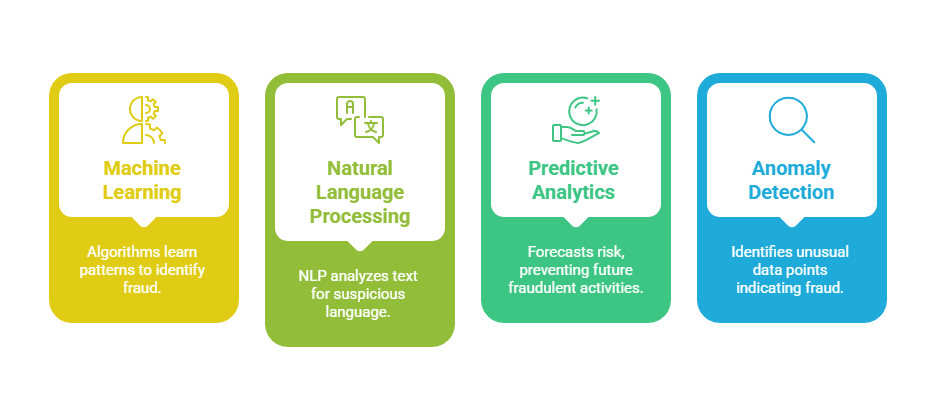
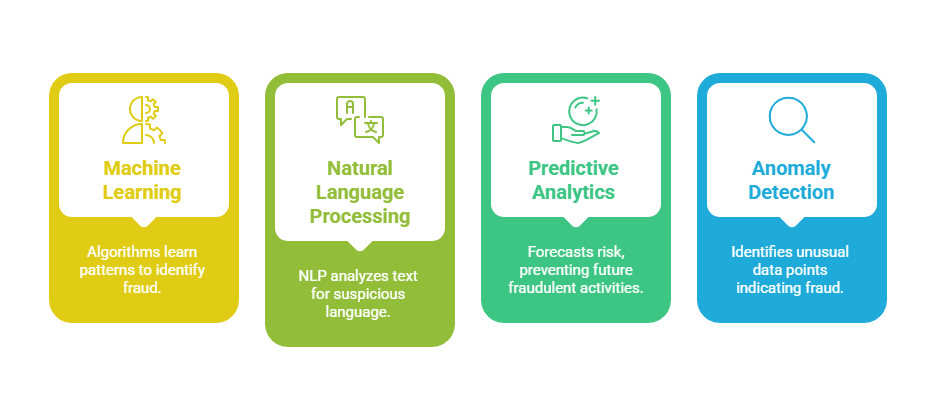
Machine Learning (ML)
Machine learning algorithms analyze vast datasets to identify patterns and anomalies that may indicate fraudulent activities. By learning from historical data, these models can detect subtle deviations from normal behavior, such as unusual billing patterns or service frequencies. For instance, ML models can flag providers who suddenly increase the volume of high-cost procedures, prompting further investigation.
Natural Language Processing (NLP)
NLP enables the analysis of unstructured data, such as clinical notes and claim descriptions. By extracting relevant information and identifying inconsistencies or unusual language patterns, NLP tools can uncover potential fraud that might be missed through structured data analysis alone.
Predictive Analytics
Predictive analytics combines statistical techniques with AI to forecast potentially fraudulent activities. By assessing risk scores and evaluating the likelihood of fraud based on historical and real-time data, healthcare payers can prioritize investigations and allocate resources more effectively.
Anomaly Detection
Anomaly detection algorithms identify data points that deviate significantly from established norms. In healthcare, this could involve detecting claims that differ markedly from typical billing patterns, signaling potential fraud. These algorithms are particularly useful in uncovering new or evolving fraudulent schemes.
By integrating these AI technologies, healthcare organizations can enhance their fraud detection capabilities, leading to more efficient operations and reduced financial losses.
5. Benefits of AI Integration in Healthcare Fraud Detection
Integrating AI into healthcare fraud detection offers several significant advantages, enhancing the efficiency and effectiveness of identifying and preventing fraudulent activities.
Real-Time Detection and Prevention
AI systems can analyze vast amounts of data in real-time, allowing for the immediate identification of suspicious claims. This proactive approach enables healthcare payers to intercept fraudulent activities before payments are disbursed, reducing financial losses and administrative burdens.
Enhanced Accuracy and Reduced False Positives
Traditional rule-based systems often generate a high number of false positives, leading to unnecessary investigations. AI’s advanced algorithms can more accurately distinguish between legitimate and fraudulent claims, minimizing false alerts and allowing investigators to focus on genuine threats.
Scalability and Efficiency
AI solutions can effortlessly scale to handle the growing volume of healthcare data, ensuring consistent protection across the healthcare system. This scalability enhances operational efficiency, enabling organizations to manage large datasets without compromising on detection capabilities.
Adaptive Learning and Continuous Improvement
AI systems employ machine learning techniques that allow them to learn from new data continuously. This adaptive learning ensures that the systems evolve with emerging fraud patterns, maintaining their effectiveness over time.
Improved Resource Allocation
By automating routine tasks and accurately identifying fraudulent claims, AI enables healthcare organizations to allocate their resources more effectively. Investigators can concentrate on complex cases, while AI handles the initial screening, leading to better utilization of human resources.
6. Challenges and Considerations in Implementing AI for Healthcare Fraud Detection
While AI offers significant advantages in detecting healthcare fraud, its implementation is not without challenges. Healthcare payers must navigate several complexities to effectively integrate AI into their fraud detection systems.
Data Quality and Integration
AI systems rely heavily on high-quality, comprehensive datasets to function effectively. However, healthcare data often exists in silos across various providers, payers, and systems, leading to fragmentation and inconsistencies. Integrating this disparate data into a unified system is a complex task that requires significant effort and resources.
Regulatory Compliance and Privacy Concerns
Handling sensitive patient data necessitates strict adherence to regulations such as the Health Insurance Portability and Accountability Act (HIPAA) in the United States. Ensuring that AI systems comply with these regulations is crucial to protect patient privacy and avoid legal repercussions.
Explainability and Transparency
AI models, particularly complex ones like deep learning algorithms, often operate as “black boxes,” making it difficult to understand how they arrive at specific decisions. This lack of transparency can hinder trust and acceptance among stakeholders. Developing explainable AI models is essential to ensure that decisions can be justified and understood by users.
Resource Constraints
Implementing AI systems requires substantial investment in technology, infrastructure, and skilled personnel. Smaller healthcare providers may find it challenging to allocate the necessary resources, potentially widening the gap between large and small organizations in fraud detection capabilities.
Evolving Fraud Tactics
Fraudsters continually adapt their methods to bypass existing detection systems. AI models must be regularly updated and trained on new data to recognize emerging fraud patterns. This ongoing maintenance is essential to ensure the continued effectiveness of AI-based fraud detection.
Addressing these challenges requires a strategic approach that includes investing in data integration solutions, ensuring regulatory compliance, developing transparent AI models, allocating sufficient resources, and maintaining continuous model updates.
7. Real-World Applications and Case Studies of AI in Healthcare Fraud Detection
The integration of Artificial Intelligence (AI) into healthcare fraud detection has yielded tangible results across various organizations. Here are some notable examples:
1. Milliman and Mastercard’s AI Collaboration
Milliman, in partnership with Mastercard’s Brighterion AI, implemented an advanced AI system to enhance their Payment Integrity (MPI) solution. This collaboration led to the identification of $239 million in fraudulent, wasteful, and abusive healthcare claims. The AI models analyzed over 90 different scenarios, including biologically implausible procedures and excessive service units, providing a significant return on investment for their clients.
2. Humana’s AI-Powered Fraud Detection
Humana, a leading health insurance company, deployed machine learning algorithms to scrutinize claims data for anomalies. Within the first year, this AI-driven approach uncovered over $10 million in potential fraudulent activities by detecting irregular billing patterns and suspicious provider behaviors.
3. Anthem’s Real-Time Use of AI in Fraud Prevention Framework
Anthem integrated AI with NLP to monitor claims in real-time. This system effectively flagged potential fraud scenarios, such as duplicate claims and improper coding. As a result, Anthem reported a 25% reduction in fraudulent payouts within six months of implementation.
5. Federal Agencies Utilizing AI for Fraud Detection
The U.S. Department of Justice (DOJ) and the Department of Health and Human Services (HHS) have expanded their use of AI and machine learning to identify healthcare fraud. These technologies analyze vast datasets to detect anomalies, such as providers billing for an implausible number of hours or services, thereby enhancing the efficiency of fraud investigations.
Conclusion
Fraud in healthcare erodes trust, slows down reimbursements, and diverts resources away from actual care. Traditional detection systems have reached their limits, especially as fraud schemes evolve in complexity. AI doesn’t offer a magic fix, but it does offer better tools, faster pattern recognition, fewer false leads, and the ability to learn and improve over time.
For healthcare payers, this shift is about giving them the insight and bandwidth to focus where it matters. As the technology matures, those who adopt AI thoughtfully — with the right data foundation, regulatory alignment, and internal buy-in — are likely to lead the charge in tackling healthcare fraud with more clarity and control.
At Nalashaa Healthcare Solutions, we help healthcare payers strengthen their fraud detection strategies through smarter technology choices that align with evolving needs, not just current challenges.
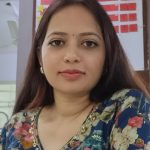
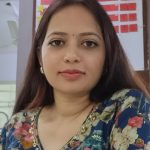
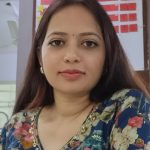
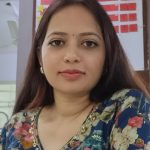
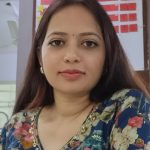
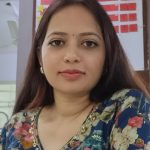
Latest posts by Priti Prabha (see all)
- The Role of AI in Fraud Detection for Healthcare Payers - April 20, 2025