[vc_row][vc_column][vc_column_text]The Importance of Predictive Models in Clinical Trials
Every year in the United States, close to 1.7 million people are diagnosed with cancer for the first time. This makes the recruitment of patients to test the efficacy of potentially life-saving experimental cancer drugs, extremely important. While the efforts from research facilities and hospitals have been laudable, there are significant challenges that continue to hamper the efficiency of clinical trials.
Understanding the Challenges in Clinical Trials
The clinical trial of a new drug is a long and expensive process. The global clinical trials market size for the year 2018 was estimated to be at $44.2 billion. Given the scale of the investment, failure is usually not an option for the clinical trials companies, medical sciences universities, and hospitals. However, the statistics about clinical trials aren’t very encouraging.
Almost 80% of clinical trials are unable to meet the required patient enrollment times. This results in a loss of close to $8M for each day the market release of the drug is delayed. The cost of every new drug that is tested on patients before the Food and Drug Administration agency (FDA) approval stands at anywhere between $161M to $2B. Along with low patient participation, the other profound causes that hamper the process of clinical trials are listed below:
- Reduced patient enrollment due to the lack of patient awareness
- Competition among clinical studies
- Rudimentary data collection methods
- Pushing the costs of individual trials onto patients
Using Predictive Models to Streamline Clinical Trials
Predictive models use many techniques ranging from Data Mining to Machine Learning (ML) and Artificial Intelligence (AI). These models find patterns in historical clinical trials data and the latest advancements in drug design to find an eligible patient for a trial. Predictive models primarily capture relationships among many factors to assess the risks. It makes this branch of data analytics well-suited to address the most profound challenges that researchers face in clinical trials.
Patient Enrollment: Predictive models extract relevant information from a patient’s medical record and compare it with the ongoing trials to suggest matching studies. By extracting information from EHRs and medical image databases, independent software vendors (ISVs) can help researchers make better predictions about patient eligibility, giving them a robust solution for their patient enrollment problems.
Medical datasets can suggest eligible patients to researchers within minutes, using predictive models, as opposed to the months that it usually takes. ISV solutions like machine learning algorithms that can be plugged into medical health records can help research labs match patients with ongoing trials that are well-suited to their needs.
Tracking Biomarkers: Biomarkers are measurable indicators of a physiological event in the human body. They can be anything from a gene, protein molecule or physiological functions such as blood pressure. Researchers track specific biomarkers to predict relevant clinical outcomes across treatments and population demographics. By obtaining standardized data sets on the effect of drugs that are tested in a clinical trial, researchers can get insights into the physiology of degenerative diseases.
Using a potent combination of wet-lab methodologies and predictive models such as ‘k-means cluster analysis’, ‘differential abundance analysis’ and ‘survival analysis’, researchers can predict genomic distributions and track the interaction of biomarker proteins in a patient’s body. This vastly helps reduce the dependency on patient-based clinical trials, especially for prototype drugs meant for rare diseases that have a perpetual genetic mutation cycle.
Master Clinical Trial Protocols (MAPs): The FDA has created the Master Clinical Trials Protocols (MAPs) to improve clinical trials efficiency and reduce the costs. MAPs is the draft guidance for the industry that is aimed at answering questions about a medical condition, faster than clinical trials. It helps researchers and doctors expedite drug development and biologics. MAPs streamline the clinical trials process by improving trials data quality and promoting the usability of this data.
With the MAPs initiative from the FDA, ISVs are in a great position to test the efficiency of their ML and AI algorithms with the data received from historical clinical trials. This also opens up the opportunity for healthcare IT companies to leverage the accuracy of clinical trial data to develop predictive models that can simulate clinical trials that surpass the efficacy of patient-based trials.
The Way Forward
The real value of clinical trials data can be harnessed effectively only with the right design of data warehousing and mining strategy. Only with competent data services that encompass the entire data life cycle can clinical trials companies establish an ecosystem that delivers precision with predictive data modeling.
Nalashaa is a healthcare IT services expert and our 10 years of experience in the domain makes us competent enough to help our clients aggregate and integrate data from a wide variety of clinical settings. Our expertise with predictive modeling, through the use of ML, AI and deep learning techniques are well suited to streamline a standard clinical trials process towards better efficiency and reduced costs.
To know more about our Healthcare IT services, reach out to us at info@nalashaa.com[/vc_column_text][/vc_column][/vc_row]
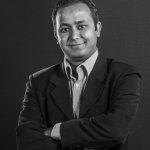
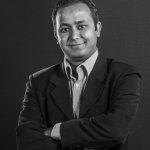
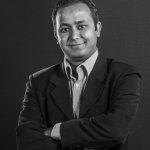
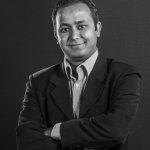