Data is the currency of modern healthcare—its value lies in transforming raw information into actionable insights that drive patient care, research, and operational excellence. Yet, the true potential of healthcare data often goes undiscovered due to pervasive quality issues. Even a single error in a patient’s EHR can lead to misdiagnoses, delays in treatment, or administrative inefficiencies.
Research reveals that inaccurate or incomplete data accounts for nearly 30% of adverse medical events, while operational inefficiencies driven by poor data quality contribute to significant financial losses. As regulatory frameworks like HIPAA demand stricter data handling and interoperability standards become prevalent, managing data quality is no longer optional—it’s essential.
This blog explores the details of Healthcare Data Quality Management (DQM), its challenges, and the strategies organizations can adopt to elevate their data practices and improve outcomes.
What is Healthcare Data Quality Management?
Healthcare Data Quality Management (DQM) is the process of ensuring health data is accurate, consistent, complete, timely, and accessible throughout its lifecycle. It is crucial in an environment where data integrity directly influences patient outcomes, operational efficiency, and compliance with regulations.
Core Components of Data Quality Management in Healthcare
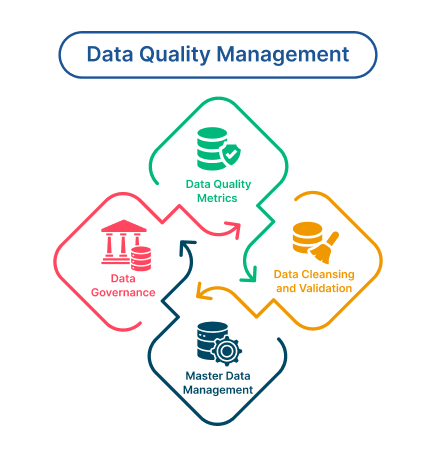
Effective healthcare data quality management is built on foundational components that ensure accuracy, consistency, and accessibility. These components show the importance of data quality in healthcare that work together to maintain the integrity of data and support reliable decision-making
1. Data Quality Metrics
Data quality metrics provide measurable benchmarks to assess and improve the reliability of healthcare data. Key metrics include:
- Accuracy: Ensuring data is error-free and reflects the true state of patient information.
- Completeness: Verifying that all necessary data points, such as patient demographics and medical histories, are captured without omissions.
- Consistency: Ensuring data remains uniform across different systems, preventing conflicts that could compromise decision-making.
- Timeliness: Making data available promptly for clinical, administrative, and operational purposes. These metrics form the foundation for identifying gaps and implementing corrective measures to enhance data integrity.
- Accessibility: Only authorized personnel should have secure and seamless access to data.
2. Data Governance
Data governance establishes policies, roles, and accountability to maintain data quality throughout its lifecycle. A robust governance framework includes:
- Policies and Standards: Clear guidelines for data collection, storage, and sharing that align with regulatory requirements like HIPAA.
- Role Definition: Assigning responsibilities for data stewardship and quality assurance to ensure adherence to established policies.
- Monitoring and Auditing: Regular audits to identify discrepancies and ensure continuous compliance with data standards. Data governance ensures consistent practices across an organization by fostering accountability and transparency.
3. Data Cleansing and Validation
Data cleansing and validation processes ensure that healthcare data remains accurate and useful by addressing errors, inconsistencies, and redundancies. Key practices include:
- Error Detection and Correction: Identifying and rectifying inaccuracies, such as duplicate entries or incorrect patient details.
- Standardization: Ensuring data adheres to standardized formats, improving interoperability across systems.
- Validation Rules: Implementing automated checks during data entry to flag and correct anomalies in time. These processes reduce the risk of errors that can compromise patient care and operational efficiency.
4. Master Data Management (MDM)
MDM consolidates data from multiple sources to create a unified and consistent view, which is essential for effective healthcare operations. Core benefits of MDM include:
- Data Unification: Integrating disparate data from EHRs, lab systems, and imaging platforms into a single, reliable source.
- Elimination of Redundancies: Reducing duplicate records and ensuring consistency across systems.
- Improved Decision-Making: Providing a comprehensive view of patient information to support informed clinical and administrative decisions. MDM enhances data usability and supports scalability as healthcare organizations expand and adopt new technologies.
The Role of Data Quality in Healthcare: Key Areas of Impact
The value of data in healthcare lies in its ability to guide decisions, improve patient outcomes, and streamline operations. However, this value depends on the quality of the data. Here’s how it influences key areas:
1. Clinical Decision-Making
Accurate and timely data is essential for effective diagnoses and treatment plans. Missing or erroneous information in EHRs—such as incomplete lab results or overlooked medical histories—can lead to misdiagnoses or unnecessary interventions. High-quality data ensures clinicians can rely on consistent and complete information to make patient-centered decisions and improve care outcomes.
2. Patient Safety
Precise data is critical for reducing medical errors and ensuring patient safety. For example, a BMC Health Services Research study found that 13% of medication records had discrepancies, many of which resulted in errors. Inaccurate records, such as conflicting allergy information, can lead to adverse drug reactions or incorrect treatments. By standardizing data entry and implementing validation tools, healthcare providers can minimize these risks and deliver safer care.
3. Operational Efficiency
Operational workflows rely on accurate data to reduce inefficiencies and cut costs. Reliable information helps organizations manage resources, streamline administrative processes, and track inventory effectively. For instance, accurate inventory data can reduce supply chain waste by identifying usage patterns and forecasting needs. Clean data also supports smoother patient scheduling and billing, eliminating bottlenecks and improving productivity.
4. Regulatory Compliance
Strict regulatory frameworks, such as HIPAA and the 21st Century Cures Act, require healthcare organizations to maintain accurate and secure data. Inconsistent or incomplete records can lead to compliance failures, exposing organizations to penalties. For example, a healthcare provider faced a $1.25 million fine in 2023 for inadequate data security. Establishing robust governance ensures compliance, protects sensitive information, and reduces the risk of financial losses or reputational harm.
5. Research and Innovation
Advancing medical research requires clean, standardized data. During the COVID-19 pandemic, researchers depended on interoperable datasets to analyze virus trends and assess vaccine safety and efficacy. Without such reliable data, delays in identifying solutions could have prolonged the crisis. Beyond emergencies, high-quality data continues to support precision medicine, population health studies, and disease modeling.
6. Strategic Planning
Strategic decision-making depends on accurate insights into patient populations, healthcare trends, and organizational performance. Clean data helps healthcare organizations to identify service gaps, manage risks, and plan for growth. For instance, analyzing demographic data helps hospitals expand service lines tailored to community needs while managing costs effectively.
Healthcare Data Quality Challenges
Managing healthcare data effectively requires addressing a range of challenges that compromise its quality. These challenges hinder clinical decision-making, operational efficiency, and regulatory compliance. Here are the most pressing issues:
1. Inconsistent Data Entry
Data entry inconsistencies arise from varied input methods, human error, and a lack of standardized protocols. For instance, differences in how patient demographics or clinical notes are recorded can lead to conflicting information across systems. This affects clinical care and complicates data analysis and reporting. Implementing standardized data entry guidelines and leveraging automation can help mitigate this issue.
2. Incomplete Patient Records
Gaps in patient records are a significant challenge, often resulting from missing information during data collection or unstructured documentation. For example, critical details like medication histories or allergies may be absent, leading to treatment delays or adverse reactions. These gaps also obstruct population health studies and compliance reporting. Regular audits and staff training in data completeness are essential to ensure comprehensive patient records.
3. Data Integration and Interoperability
Healthcare organizations often rely on multiple systems, such as EHRs, lab systems, and imaging software, which may not communicate effectively. The lack of interoperability results in siloed data that cannot be easily shared or consolidated. This is particularly problematic in care transitions, where fragmented data can delay treatment. Adopting interoperability standards (HL7, FHIR) is key to creating seamless data exchange across systems and organizations.
4. Duplicate and Fragmented Data
Duplicate and fragmented records occur when patient information is stored across multiple systems without proper linkage or deduplication efforts. For example, a patient might be registered multiple times under slightly different names or identifiers, creating redundant records. These discrepancies complicate care delivery and lead to inefficient resource allocation. Master Data Management solutions and advanced deduplication tools can address these challenges, ensuring a unified patient view.
Key Technologies Supporting Data Quality in Healthcare
Technology is directly responsible for addressing data quality challenges of healthcare organizations. Here are the key technologies driving improvements:
1. Interoperability Standards
Interoperability standards like HL7 and FHIR enable seamless data exchange between disparate systems. These standards provide:
- Consistent Data Formats: Uniformity in how patient information is stored and shared across EHRs, labs, and imaging systems.
- Efficient Care Transitions: Allowing healthcare providers to access complete patient histories regardless of the system used. For example, implementing FHIR APIs can streamline communication between providers, reducing delays in accessing critical data.
2. AI and Machine Learning
AI-powered tools are transforming data quality management by automating error detection and improving decision-making. Key applications include:
- Anomaly Detection: AI can identify discrepancies in patient records, such as mismatched medication dosages or conflicting diagnoses.
- Predictive Analytics: Machine learning models can predict data inconsistencies or identify areas needing validation, reducing downstream errors.
3. Data Quality Management Tools
Specialized tools like SAS data management or information, provide comprehensive solutions for managing data quality, including:
- Validation and Cleansing: Automatically identifying and correcting inaccuracies in time.
- Deduplication: Detecting and merging duplicate records to ensure a single source of truth. For example, tools like Informatica and Talend are widely used to streamline data cleansing and validation processes in large healthcare systems.
These tools often incorporate automation, machine learning algorithms, and built-in interoperability standards to ensure accurate and consistent data across EHRs, lab systems, and other healthcare applications.
4. Data Auditing Software
Regular data audits are essential for maintaining accuracy and compliance, and auditing software simplifies this process:
- Compliance Monitoring: Tools help ensure data adheres to regulatory standards such as HIPAA and the 21st Century Cures Act.
- Trend Analysis: Auditing software provides insights into recurring errors, helping organizations address root causes. These tools reduce the manual burden of audits, ensuring consistent oversight and proactive improvements.
Strategies for Effective Data Quality Management
Data quality is a continuous process and isn’t a one-time fix. It requires a deliberate, ongoing approach tailored to the unique complexities of healthcare systems. Here are actionable strategies to address common challenges:
1. Standardizing Data Entry Protocols
Consistency in data entry reduces errors and ensures uniformity across systems. Key practices include:
- Developing Standard Templates: Predefined forms and fields minimize variability in how data is recorded.
- Training Staff: Regular training programs for clinical and administrative staff ensure adherence to standardized protocols.
- Automation: Leveraging dropdown menus and auto-fill options reduces manual errors during data input.
2. Implementing Regular Data Audits
Frequent audits help identify and rectify inconsistencies, ensuring data remains reliable over time:
- Proactive Issue Identification: Audits uncover missing or incorrect data, enabling corrective action before errors impact patient care.
- Compliance Checks: Adherence to regulatory frameworks like HIPAA through scheduled reviews.
- Using Auditing Tools: Automated tools streamline the audit process, reducing manual efforts and improving accuracy.
3. Data Integration Solutions
Integrating disparate systems ensures a unified view of data across the organization:
- Adopting Interoperability Standards: Implementing HL7 and FHIR enables seamless communication between systems, reducing data silos.
- Data Warehousing: Consolidating information from EHRs, labs, and imaging systems into a centralized repository improves accessibility and consistency.
- APIs for Real-Time Exchange: APIs allow live data flow such that clinicians have access to the latest patient information.
4. Managing Patient-Generated Data
With the rise of wearable devices and remote monitoring tools, patient-generated data presents new challenges:
- Validation Processes: Implementing validation checks ensures that data from wearables and apps is accurate and actionable.
- Integration into EHRs: Combining patient-generated data with existing clinical records provides a holistic view for informed decision-making.
- Patient Education: Educating patients on the importance of accurate data entry, especially in apps and portals, enhances the quality of self-reported information.
Case Studies: Real-World Benefits of High-Quality Data
The stakes in healthcare are far too high for data errors to go unnoticed. From life-saving clinical decisions to the effective management of resources, data quality is the backbone of modern care delivery. Yet, many organizations struggle to overcome gaps in accuracy, integration, and accessibility. The following real-world examples showcase how healthcare providers and networks have successfully tackled these challenges, turning data into a powerful enabler of better outcomes and operational excellence.
1. Enhancing Patient Safety Through Accurate Data Management
Case Study: Yale New Haven Hospital
Yale New Haven Hospital recognized the critical role of accurate data in patient safety. By redesigning their neurology and neurosurgery intensive care units, they enabled direct communication between care providers, ensuring that accurate and timely patient data was available at the point of care. This initiative led to improved patient outcomes and enhanced safety protocols.
2. Improving Operational Efficiency via Data Integration
Case Study: Idaho Health Data Exchange (IHDE)
The IHDE collaborated with 4medica to transform its data portal into a stakeholder-oriented ecosystem. This transformation focused on data completeness, reliability, and quality, with a strong emphasis on identity management. As a result, IHDE improved data accuracy and operational efficiency, facilitating better healthcare delivery across the state.
3. Advancing Population Health Management with Predictive Analytics
Case Study: Kaiser Permanente and IBM Watson Health
Kaiser Permanente partnered with IBM Watson Health to improve population health management through predictive analytics. This collaboration enabled the organization to identify high-risk populations and implement targeted interventions, leading to improved health outcomes and more efficient resource utilization.
Future Trends in Healthcare Data Quality
The demand for high-quality data in healthcare is on the rise, driven by the increasing complexity of care delivery, expanding patient datasets, and evolving regulations. Organizations no longer just aim to “fix” data issues—they’re now looking to predict and prevent them. As new technologies and practices emerge, the future of healthcare data quality management is shifting toward automation, real-time solutions, and a stronger emphasis on collaboration and interoperability. Let’s explore the key trends shaping this transformation.
1. AI-Driven Data Quality Management
Artificial intelligence and machine learning are changing how data quality issues are identified and resolved. AI-driven systems can
- Detect Anomalies: Flag inconsistencies in patient records or medication orders before they affect care delivery.
- Automate Data Cleansing: Identify and rectify errors across large datasets with minimal human intervention.
- Enhance Predictive Analytics: Support proactive care management by analyzing high-quality data for risk factors and health trends. As AI tools become more sophisticated, their role in ensuring data accuracy and consistency will continue to grow.
2. Value of Patient-Generated Data
Wearables, mobile apps, and remote monitoring devices are flooding healthcare systems with valuable patient data. Beyond monitoring vitals, this explosion of patient-generated data offers new opportunities:
- Proactive Interventions: Continuous data streams from wearables can show early warning signs for better care decisions.
- Personalized Care Plans: Insights from daily activity and health patterns allow clinicians to tailor treatment for chronic conditions like diabetes or hypertension.
- Advanced Analytics: When integrated with clinical systems, patient-generated data enhances predictive analytics, improving resource planning and care delivery.
Patient-generated data, when validated and integrated securely, is redefining care by connecting real-world patient behavior with clinical insights.
3. Continuous Data Quality Monitoring and Automation
Traditional periodic audits are giving way to continuous monitoring systems that provide real-time insights into data quality. Key advancements include:
- Monitoring Tools: Implementing systems that automatically flag discrepancies or missing data points during operations.
- Workflow Automation: Reducing manual efforts in error detection and correction through integrated quality checks.
4. Interoperability Advancements
With the widespread adoption of interoperability standards healthcare organizations are moving closer to seamless data exchange. Future developments may include:
- Global Standards Alignment: Coordinating data-sharing protocols across international healthcare systems for better collaboration.
- Interoperable Analytics: Enabling real-time analytics on interoperable datasets to support faster and more accurate decision-making. These advancements are essential for creating unified and actionable datasets across healthcare networks.
5. Data Quality as a Service (DQaaS)
The rise of cloud-based solutions is making Data Quality as a Service (DQaaS) a practical option for healthcare providers. These services offer:
- Scalability: Adapting data management capabilities to organizational growth without the need for extensive infrastructure investments.
- Expert Support: Leveraging external expertise for complex data quality challenges.
- Cost Efficiency: Reducing overheads associated with on-premises systems. DQaaS is expected to play a significant role in democratizing access to advanced data quality tools.
How Nalashaa Can Help
Healthcare’s reliance on data will only continue to grow, with its quality determining the success of patient care, research, and operational efficiency. Addressing challenges like inconsistent data entry, incomplete records, and interoperability requires more than tools.
At Nalashaa, we understand the importance of healthcare data. With 13+ years of experience in healthcare IT solutions, we help organizations implement robust data quality management systems tailored to their unique challenges. Our expertise spans:
- Interoperability Solutions: Integrating systems using standards to enable real-time data sharing and eliminate silos.
- Custom Data Governance Frameworks: Designing policies and workflows to ensure compliance with HIPAA, the 21st Century Cures Act, and other regulatory frameworks.
- EHR Optimization and Analytics: Ensuring accuracy and usability of electronic health records for better clinical and operational decisions.
Ready to transform your data quality management practices? Let’s work together to create a healthier, data-driven future.
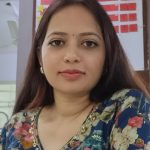
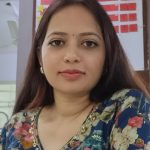
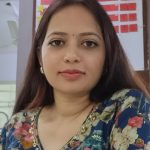
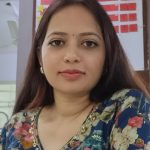
Latest posts by Priti Prabha (see all)
- Data Governance Regulations in Healthcare (2025) - January 9, 2025