Artificial intelligence (AI) has made significant strides in revolutionizing the healthcare industry, particularly in diagnosing diseases, predicting patient outcomes, and streamlining treatment plans. The healthcare industry is already familiar with the benefits of data analytics in predictive diagnosis, and AI is increasing this scope manifolds. However, as AI continues to penetrate the realm of healthcare, controversial questions arise, particularly in end-of-life decision-making. This blog post delves into the ethical dilemmas surrounding the role of AI in making crucial decisions that affect patient’s life and the benefits of it when utilized in a controlled manner.
Evolution of AI and Its Introduction to Healthcare
Early Concepts and Development (1950s-1960s)
- 1950s: The journey of AI begins with pioneers like Alan Turing, whose “Turing Test” proposed evaluating a machine’s intelligence by its indistinguishability from human intelligence.
- 1960s: Foundational AI concepts like problem-solving and symbolic reasoning take shape, laying the groundwork for future developments.
Rule-Based Systems and Expert Systems (1960s-1970s)
- 1960s-1970s: The era saw the advent of rule-based systems, where AI used explicit rules to emulate human expertise in narrow domains, evolving into expert systems designed to solve complex problems via if-then rules, though they struggled with uncertainty and learning.
Machine Learning and Neural Networks (1980s-1990s)
- 1980s-1990s: A pivotal shift toward machine learning, with systems learning from data and improving over time. Neural networks, inspired by the human brain’s structure, emerge, despite being limited by computing power and data scarcity.
Rise of Big Data and Deep Learning (2000s-Present)
- 2000s: The big data era ushers in a critical mass of data, enabling deep learning’s rise. Deep learning, a machine learning subset, leverages layered neural networks to extract complex data features, achieving remarkable success in image and speech recognition.
Integration of AI Across Industries (Present)
- Present: AI’s influence expands across various sectors, notably healthcare, where it’s reshaping disease diagnosis, treatment personalization, clinical testing, and enhancing patient engagement. AI’s capability in genomic medicine and drug discovery marks a significant leap forward, contributing to outbreak surveillance and public health.
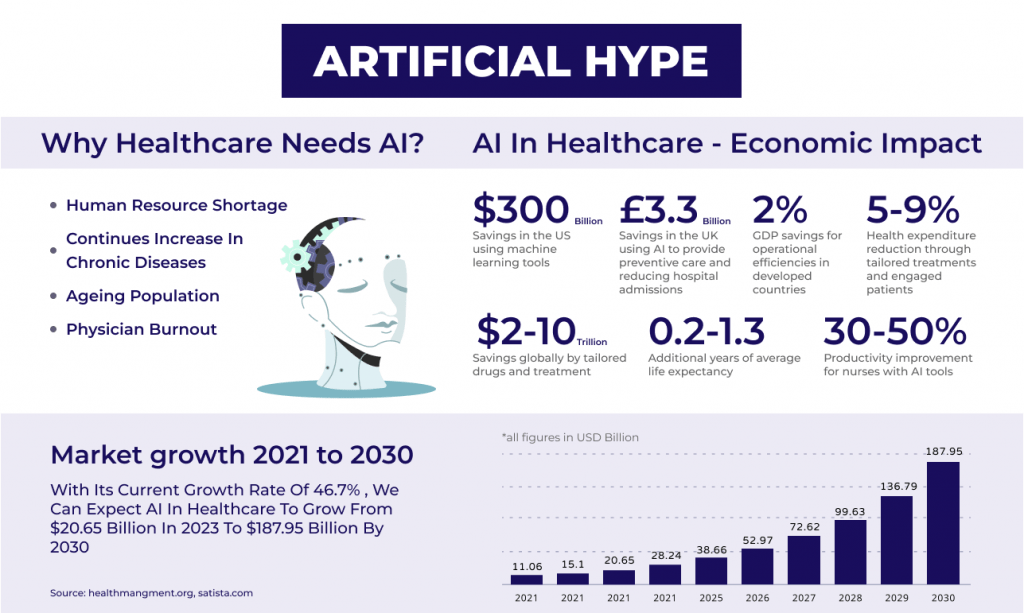
The CAGR of AI in the global healthcare market is soaring at an impressive rate, estimated at around 48% for the forecast period of 2024-2029. This remarkable growth translates to a significant increase in the adoption of AI technologies, with the market size expected to reach $148.4 billion by 2029.
Types of Artificial Intelligence in Healthcare
The spectrum of AI technologies enhancing healthcare is broad, encompassing several key forms that each bring unique capabilities to the medical field:
Machine Learning (ML)
Machine Learning, a subset of AI, involves the development of algorithms that can learn and make predictions or decisions based on data. In healthcare, ML is a game-changer for several reasons:
- Predictive Analytics: ML algorithms can predict disease outbreaks, patient admissions, and the likelihood of diseases based on historical data, improving public health surveillance and hospital resource management.
- Diagnostics: Advanced ML models, particularly deep learning, are used to analyze medical images like X-rays, MRIs, and CT scans with high accuracy, assisting in early disease detection and reducing diagnostic errors.
- Personalized Medicine: ML enables the analysis of vast datasets, including genetic information, to tailor treatments to individual patients. This approach improves treatment outcomes by considering each patient’s unique health profile.
Natural Language Processing (NLP)
NLP allows computers to understand and interpret human language, making it a vital tool in healthcare for:
- Clinical Documentation: Automating the transcription and organization of clinical notes and reports, saving healthcare professionals time and reducing manual errors.
- Information Extraction: Extracting relevant information from unstructured data sources such as patient records, research papers, and clinical trial reports. This capability supports research and helps clinicians stay informed about the latest treatments and guidelines.
- Patient Interaction: Enhancing patient engagement through chatbots and virtual assistants that provide health advice, medication reminders, and support for routine inquiries, improving the healthcare experience.
Robotic Process Automation (RPA)
RPA uses software robots to automate repetitive and rule-based tasks, which in healthcare translates to:
- Administrative Efficiency: Automating tasks such as appointment scheduling, patient registration, billing, and claims processing. This reduces operational costs and allows staff to focus on more critical aspects of patient care.
- Data Management: Streamlining the management of patient records, including data entry, updates, and retrieval. This ensures the accuracy and accessibility of patient information, enhancing care coordination.
- Physical Robots: Robots in healthcare extend beyond digital algorithms to include physical machines that interact with the real world.
- Surgical Robots: Enhancing surgical precision and allowing minimally invasive procedures. Surgeons control robotic arms with high precision, improving patient outcomes and reducing recovery times.
- Service Robots: Performing logistical tasks within hospitals, such as delivering medications, supplies, and even sterilizing rooms. These robots improve efficiency and reduce the workload on human staff.
Combined Technologies
In practice, these technologies often work together, creating synergies that amplify their benefits. For instance, NLP can enhance ML models by structuring unstructured data, which ML algorithms can then learn from. Similarly, RPA and physical robots can incorporate ML and NLP to become more adaptive and intelligent, handling more complex tasks and interactions.
Deep Learning
Deep Learning is a subset of machine learning that utilizes neural networks with many layers. It is particularly powerful for:
- Advanced Imaging Analysis: Deep learning excels in interpreting complex patterns in medical imaging, significantly improving the diagnosis of diseases from cancer to neurological disorders.
- Drug Discovery and Development: Speeding up the process of identifying potential therapeutic drug candidates by predicting molecular behavior and drug interactions more efficiently than traditional methods.
Computer Vision
Computer Vision enables computers to derive meaningful information from digital images, videos, and other visual inputs, used extensively in:
- Radiology: Automating the analysis of X-rays, MRIs, and CT scans to detect abnormalities such as tumors, fractures, or diseases at an early stage.
- Pathology: Enhancing the examination of biopsy samples, helping pathologists identify disease markers with higher precision and speed.
Predictive Analytics
Beyond its foundation in machine learning, predictive analytics in healthcare specifically refers to the use of data, statistical algorithms, and machine learning techniques to identify the likelihood of future outcomes based on historical data:
- Risk Assessment: Identifying patients at high risk of developing certain conditions, allowing for early intervention or personalized care plans.
- Resource Allocation: Helping hospitals predict patient inflow and manage staffing and resources more effectively to enhance patient care while optimizing operational costs.
Genomics and Precision Medicine
The application of AI in genomics involves analyzing genetic data to understand diseases and individual responses to treatment, facilitating:
- Genetic Risk Scoring: Predicting an individual’s predisposition to diseases based on their genetic makeup, enabling preventive measures or targeted surveillance.
- Personalized Treatment Plans: Tailoring medical treatments to individual genetic profiles, especially in oncology, to improve treatment efficacy and reduce side effects.
Internet of Medical Things (IoMT)
The IoMT refers to the network of medical devices and applications that connect to healthcare IT systems through online computer networks. AI enhances IoMT by:
- Remote Monitoring: Enabling continuous monitoring of patients’ health metrics outside of conventional clinical settings, improving chronic disease management and preventive care.
- Wearable Technology: Analyzing data from wearable devices to provide real-time insights into a patient’s health status, activity levels, and potential health risks.
Chatbots and Virtual Health Assistants
These AI applications interact with patients directly, offering:
- Symptom Checkers: Providing initial consultation based on symptoms described by the patient, guiding them to appropriate care resources.
- Mental Health Support: Offering therapeutic interactions for mental health support, including cognitive behavioral therapy techniques, stress management, and more.
The Use of Artificial Intelligence in Healthcare
- Medical Imaging and Diagnostics: AI has shown remarkable potential in medical imaging and diagnostics, improving accuracy and efficiency. Machine learning algorithms can analyze medical images such as X-rays, MRIs, and CT scans to detect abnormalities, assist in early disease detection, and aid radiologists in making more accurate diagnoses.
- Precision Medicine: AI plays a crucial role in advancing precision medicine, tailoring treatments to individual patients based on their genetic makeup, lifestyle, and other relevant factors. Machine learning algorithms can analyze vast genomic datasets, identify genetic markers associated with diseases, and predict individual patient responses to specific treatments.
- Virtual Assistants and Chatbots: AI-powered virtual assistants and chatbots are transforming patient engagement and support. These tools can provide 24/7 personalized assistance, answer frequently asked questions, offer symptom assessments, and even schedule appointments.
- Drug Discovery and Development: The process of drug discovery and development is complex, time-consuming, and costly. AI accelerates this process by analyzing vast datasets and identifying patterns that may lead to the discovery of new drugs or the repurposing of existing ones. Machine learning algorithms can analyze molecular structures, predict drug-target interactions, and optimize drug design.
- Remote Patient Monitoring: AI enables remote patient monitoring, revolutionizing the way healthcare is delivered. Connected devices, wearables, and sensors collect real-time patient data, which is then analyzed by AI algorithms. This technology allows healthcare professionals to monitor patients’ vital signs, detect anomalies, and provide timely interventions, even from a distance.
- Predictive Analytics and Patient Risk Stratification: AI-driven predictive analytics enables the identification of high-risk patients and the early detection of potential health complications. By analyzing patient data, including electronic health records, medical histories, and social determinants of health, AI algorithms can identify patterns and risk factors associated with specific conditions.
- Robot-Assisted Surgery: These combine the precision of robotic systems with the expertise of surgeons. AI algorithms guide robotic surgical systems, enhancing surgical precision, and minimizing the risk of complications. Surgeons can benefit from improved visualization, increased dexterity, and augmented decision support during complex procedures.
AI Benefits in Healthcare
While ethical concerns surround the use of AI in healthcare, it is essential to acknowledge the potential benefits that AI can offer through the applications mentioned above. All of the above applications will give birth to a plethora of benefits. Some of them are listed below.
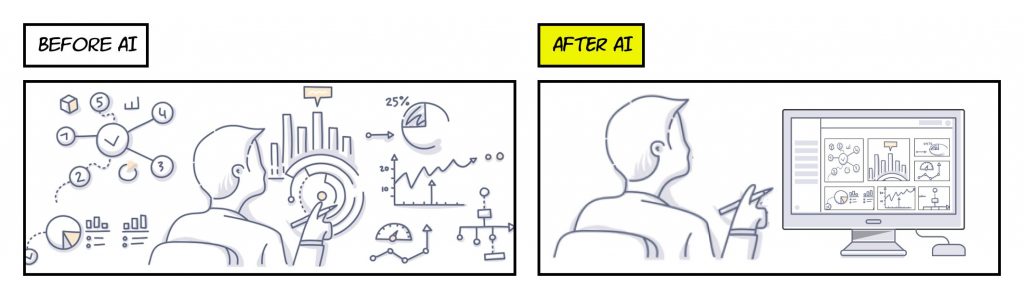
Streamlining Administrative Workflows
- Reducing Clerical Burden: AI and automation technologies are pivotal in alleviating the administrative load on healthcare workers. An alarming 25% of a US nurse’s work time is consumed by regulatory and administrative tasks. By automating routine tasks such as paperwork, AI frees healthcare professionals to focus more on patient care and less on administrative duties.
Enhancing Patient Interaction
- Chatbots for Patient Engagement: AI-powered chatbots and virtual assistants offer round-the-clock support for patient inquiries, medication guidance, and appointment scheduling. Despite concerns over data privacy and the handling of complex health conditions, these tools represent a significant step toward more responsive and accessible patient care.
- Machine Learning in Claims Management: By employing machine learning for probabilistic data matching across various databases, healthcare providers can more accurately identify and correct billing and coding errors. This capability is invaluable in ensuring the accuracy of millions of claims, saving time, and reducing financial waste for insurers, providers, and patients alike.
Operational Improvements
- Generative AI in Clinical Documentation: Generative AI assists clinicians with note-taking and content summarization, ensuring medical records are comprehensive and up-to-date. This technology aids in accurate coding and facilitates seamless information sharing across departments.
- Virtual Nursing Assistants: Aiding in medication queries and report forwarding, virtual assistants alleviate the administrative load on clinical staff, enabling them to dedicate more time to direct patient care.
Patient Safety and Fraud Prevention
- Dosage Error Reduction: AI tools monitor medication administration, flagging potential errors and ensuring patient safety. For instance, AI systems can detect inconsistencies in how patients self-administer insulin, reducing the risk of improper dosing.
- AI-Enabled Robots in Surgery: Robots equipped with AI enhance surgical precision, minimize the risk of infection, and expedite patient recovery by performing less invasive procedures.
- Combating Fraud: AI’s pattern recognition capabilities are instrumental in identifying fraudulent activities within healthcare, safeguarding financial resources, and maintaining the integrity of healthcare services.
Unifying Healthcare Data
AI excels in consolidating and analyzing patient data, offering insights that can transform disease management and treatment strategies. From managing diabetes with wearable technology to innovating drug safety processes, AI is making data more accessible and actionable for healthcare providers.
Statista’s analysis suggests a significant surge in the AI healthcare market, growing from a valuation of $11 billion in 2021 to an anticipated $188 billion by 2030. Despite this, some ethical dilemmas and other challenges are looming over everyone. Although these dilemmas are not as frightening as “The Terminator” it still raises concerns about privacy and patient consent.
Key Challenges of using AI in Healthcare
Addressing Key Challenges
Data Privacy and Security: With AI systems processing vast amounts of personal health information, the imperative for stringent data privacy and security measures cannot be overstated. Safeguarding sensitive patient data against misuse and cyber threats is paramount to maintaining trust and integrity in healthcare AI applications.
Patient Safety and Accuracy: The reliability of AI systems in providing accurate diagnoses and treatment recommendations hinges on their ability to discern complex patterns within medical data. This necessitates advanced algorithm training and seamless integration with existing healthcare IT infrastructures, ensuring that AI tools complement rather than complicate medical workflows.
Physician Acceptance and Regulatory Compliance: For AI in healthcare to reach its full potential, winning the trust of medical practitioners and ensuring adherence to federal and international regulations is critical. Transparency in AI decision-making processes and aligning AI applications with current medical standards and practices will be key to achieving widespread acceptance among healthcare professionals.
Navigating Ethical Implications
The shift towards AI-driven healthcare decisions brings forth profound ethical considerations. Issues of accountability, transparency, and the maintenance of patient privacy and autonomy are at the forefront. Particularly challenging is the issue of transparency, as many AI algorithms, especially those based on deep learning, offer little in the way of interpretable decision-making processes. This opacity poses significant dilemmas when patients seek explanations for AI-driven diagnoses or treatment plans.
Moreover, the potential for AI systems to err in patient diagnosis and treatment underscores the critical need for establishing clear lines of accountability. Compounding these concerns is the risk of algorithmic bias, which could inadvertently perpetuate disparities in healthcare outcomes based on gender, race, or other non-causal factors.
Forward Path: Ethical Governance and Multidisciplinary Solutions
Addressing the challenges and ethical dilemmas posed by AI in healthcare demands a concerted effort from healthcare institutions, regulatory bodies, and the AI community at large. Establishing governance mechanisms and ethical frameworks that prioritize patient safety, data privacy, and transparency will be essential. This endeavor requires not only continuous vigilance but also a multidisciplinary approach that bridges technology, medicine, and ethics.
To surmount the data-related challenges inherent in AI applications, innovative data annotation methods, the development of robust AI models, and close collaboration between computer scientists and healthcare practitioners are imperative. Furthermore, ensuring the ethical deployment of AI in healthcare will involve fostering an environment of ethical awareness among all stakeholders, emphasizing the importance of accountability and informed consent.
Educating the Next Generation of Healthcare Professionals
As AI becomes increasingly embedded in healthcare practices, updating medical curricula to include AI and its ethical implications will be crucial. Empowering future healthcare professionals with knowledge about AI technologies and their applications ensures a workforce capable of integrating AI tools into clinical practice thoughtfully and ethically.
Legal and Ethical Frameworks for AI in Healthcare
With the growing reliance on AI and big data, the importance of robust legal protections for patient data has never been greater. Legislation like the Health Insurance Portability and Accountability Act (HIPAA) in the U.S. and the General Data Protection Regulation (GDPR) in the EU represent steps towards safeguarding individual privacy. Yet, the dynamic nature of AI and ongoing advancements in technology call for continuous updates to these legal frameworks to address emerging challenges effectively.
Strategies to Address these Challenges
The statistics that we have at hand regarding the adoption of AI in the US healthcare ecosystem tell us that no matter the challenges AI is here to stay. Last year the
To address challenges in AI adoption, several strategies can be employed to facilitate a smoother and more effective integration of AI technologies into various industries and business processes. Here are some of the main strategies:
Education and Training Programs: Implement comprehensive education and training programs to upskill employees and stakeholders on AI technologies, their benefits, and best practices for implementation. This can help demystify AI and build confidence in utilizing its capabilities.
Ethical and Regulatory Frameworks: Develop clear ethical guidelines and robust regulatory frameworks to govern the responsible and ethical use of AI. This includes addressing privacy concerns, algorithm biases, and data security to build trust among consumers and businesses.
Collaboration and Partnerships: Foster collaboration between AI developers, industry experts, and end-users to co-create AI solutions tailored to specific needs and challenges. Partnerships can accelerate innovation and bring diverse perspectives to the table.
Change Management and Leadership Support: Implement effective change management strategies and garner strong leadership support to drive cultural shifts within organizations. This involves aligning AI adoption with strategic business goals and creating a supportive environment for experimentation and learning.
Data Quality and Accessibility: Prioritize data quality, integrity, and accessibility to ensure that AI systems have access to reliable and relevant data for training and decision-making processes. This involves data governance, quality assurance, and data-sharing initiatives where appropriate.
Interdisciplinary Teams and Skills Diversity: Form interdisciplinary teams with a diverse set of skills, including data scientists, domain experts, ethicists, and user experience professionals. This approach can bring different perspectives to problem-solving and enhance the robustness of AI solutions.
Piloting and Prototyping: Encourage the piloting and prototyping of AI applications in controlled environments to assess their feasibility, impact, and potential challenges. This iterative approach allows for refinement before full-scale deployment.
Transparency and Explainability: Emphasize the importance of transparency and explainability in AI decision-making processes. This involves making AI models interpretable and understandable to end-users, regulators, and stakeholders to build trust and mitigate risks.
Continuous Monitoring and Evaluation: Establish mechanisms for continuous monitoring, evaluation, and feedback loops to track the performance of AI systems post-implementation. This facilitates ongoing improvements, addressing emerging challenges, and ensuring alignment with organizational objectives.
By implementing these strategies, organizations can overcome the challenges associated with AI adoption and unlock the full potential of AI technologies to drive innovation, efficiency, and value creation.
To AI or Not To AI
The integration of AI in end-of-life decision-making presents a complex ethical landscape. Balancing patient autonomy, mitigating bias, considering emotional factors, and ensuring transparency and accountability are crucial in harnessing the potential benefits of AI. As the healthcare industry continues to adopt AI technologies, it is essential to engage in ongoing discussions, implement rigorous oversight mechanisms, and involve patients, healthcare professionals, ethicists, and policymakers in shaping the responsible and ethical use of AI. Ultimately, our goal should be to utilize AI as a valuable tool that enhances the idea of value-based care.
Connect with us to build a better tomorrow. Info@nalashaa.com
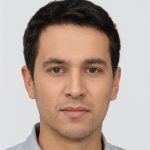
Samuel Thomas
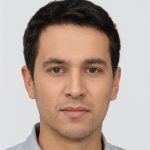
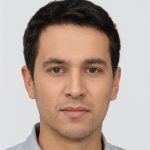
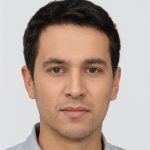
Latest posts by Samuel Thomas (see all)
- Top Regulatory Changes in Healthcare, 2023 - July 7, 2023
I genuinely appreciate the effort and dedication you put into researching and crafting this blog post. Your writing style was engaging and accessible, allowing readers like myself to grasp the complexities of AI in healthcare without feeling overwhelmed. Your insights have expanded my knowledge and sparked a keen interest in further exploring this rapidly evolving field.
Thank you once again for sharing your expertise and shedding light on the scope and benefits of AI in healthcare, as well as the ethical dilemmas that need careful consideration. I am grateful for the opportunity to learn from your well-informed perspective.