Researchers at University of Washington Tacoma have developed a machine-learning predictive analytics tool that forecasts with 82 percent accuracy the probability a patient will be readmitted within 30 days. In 2011, the federal government estimated that 30-day Medicare readmissions for all causes cost a whopping $26 billion annually, with $17 billion being attributed to avoidable events. So how can payer emphasize on improving provider services for a better care?
Healthcare finance departments need to better their systems in the face of value or quality based reimbursements, which are to take effect as of 2018. According to a recent survey by KPMG’s Healthcare & Life Sciences Practice, many respondents said that the finance departments need predictive modeling (30 percent) and analytical tools (27 percent) to successfully make the transition.
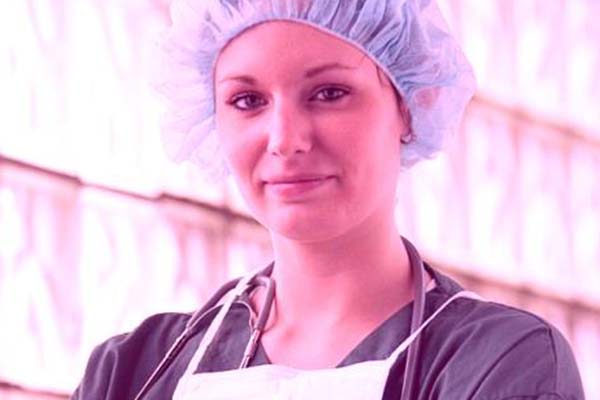
Provider incentive payout, linked to quality of care, can also be derived through healthcare payer analytics. Incentive payouts increase when patient re-admission is at a minimum. With a dedicated healthcare analytics platform for payers, they can identify and intimidate providers of possible complications, hence avoiding the re-admissions and ensuring incentive payouts. Some payers are also looking at an alternative payment program with providers, on condition that the clinical data integration is complete and healthcare data analytics can be performed.
Herein, lies the major concern for payers.
Payer technology systems are not conceived to integrate clinical data into them, and further analyze and perform predictive modeling to derive actionable data from them. Such analyzes, if done, can help in understanding Potentially Avoidable Complications (PAC), and hence gain better control on pricing and claims.
So, from a technology standpoint, lets look at the bottlenecks, which come up in the payer- provider collaboration.
- Integrating healthcare data
- Most payer systems do not have the capability to aggregate and leverage the clinical data. Payer systems need to go through an overhaul to ensure workflows have the ability to accept incoming clinical EHR information and to derive meaning from it
- Interoperability, linking multiple data sources for benchmarking and ability to acquire patient data directly and de-identify it to generate knowledge and ensure trustworthiness of the data
- Current manual aggregation of data
- In the current technology set up, there are various transactional systems that are silos of information. Each of them has their own manual conversion of available clinical data from claim forms that need not be consistent with the information recorded at the Point of Care. For a complete integration of EHR data, the technology platform should be capable of accepting data of different types (imaging files, genomic files, telemetry files) and from varying sources (RIS, Billing System etc).
- Quality of Data
- This is one aspect where healthcare payers have been very particular in their data, following industry standards and clinical terminologies. The providers on the other hand have been struggling with ensuring all their systems adhere to industry standards.
In our experience, integration of systems requires a defined plan and a clear understanding of the underlying systems involved. There are many differences in a payer and provider system, and understanding these enable us to chart a smooth integration plan and delivering success.
A step-by-step approach to healthcare payer data analysis:
- Integration of Data
- There are many systems, which will provide the required clinical data for the payer systems. Amongst these systems, the EHR would provide a large chunk of data to populate patient information, and this being the primary source of clinical data, integration must be carried out understanding all critical use cases.
- Tools which will extract, aggregate and normalize data from different sources, for use in a single application
- Analysis of Data
- Define business requirements and understand the data available to further derive meaningful information from the integrated data
- Build a reporting platform that will use the integrated data to give detailed analysis of patient disease history and further make predictions to aid in payer marketing.
Healthcare analytics tools can also help anticipate possible diseases based on population standards and help the providers assess patients for possible associated diseases. This will further reduce costs at later, more aggravated stages of the disease and an effective evidence-based-medicine.
To know more about how we can help you integrate your systems to receive clinical data, please contact us.
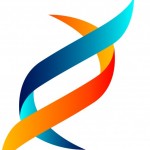
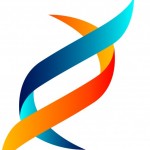
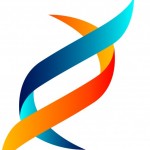
admin
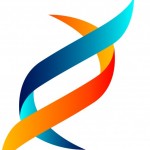
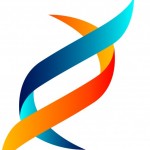
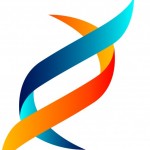
Latest posts by admin (see all)
- Happy Women’s Week! - March 10, 2017