Today, data is everywhere and in many forms. The processing power to crunch huge amounts of data and get analytical insights is exponentially increasing. Cloud technologies are boosting the effective utilization of data at a rapid rate. Low-cost storage and on-demand scalability are taking data handling capabilities to new heights. But there is a flip side to the opportunities in data analytics. Without high data quality, the pursuit for business insights trough analytics can be inefficacious.
Data Analytics & AI Solutions are stimulating meaningful insights and crafting business values. As the usage of social media apps are increasing day by day, due to new technologies and tools, complexities while capturing raw data are also increasing. The nature of data itself is changing in such a way that non-traditional (i.e. NoSQL) concepts are taking over from conventional/established standards. But as the concept “garbage in garbage out” goes, it is critical to capture and manage data adequately at the point of generation itself.
The common reasons for inadequate data are:
- Misalignment of data collection process relative to business needs
- Technical challenges at collection from multiple mediums/languages
- Human error at manual data entry
- Ambiguous guidelines on data collection
- Duplication of information
The right data governance policies aligned with business processes can reduce and eliminate low data quality. Data Governance pertains to people, processes, and technologies required to manage and protect data assets. Data Governance policies depict roles and responsibilities related to people and technologies to become gatekeepers for data input and output stages. To achieve better data quality, defined policies should cover all data systems with well-defined standards and communicate exceptions. For example, certain data elements related to a member are mandatory in Healthcare Payers, regardless of the system referenced.
Another major contributor to low quality is the ambiguous nature of business process definitions. As data is captured or generated while performing a business process, the procedures should be stable, clearly defined, and executed with consistency.
Achieving prime data quality, is a paramount task. Although, in the earliest stages, it may not be possible to have the targeted data quality, there will still be continuous requirements to process & utilize data. So, the possibility of data errors and incompleteness should be clearly defined and communicated.
Gartner estimated poor data as the primary reason for 40% of all business initiatives failing to achieve their targeted benefits.
Want to transition from ICD 9 to ICD 10?
Data Warehouse vs Data Lakes
Once individual systems, such as claims, customer support, member enrolment and eligibility attain the desired data quality levels, the next step will be to settle on a central repository, i.e. either a data warehouse (DW) or data lake (DL), based on business needs.
A data warehouse is a database optimized to analyze relational data from transactional structures and the business application line. The data structure and schema are specified in advance to optimize for quick SQL queries, where the results are usually used for operational reporting and analysis.
A data lake, on the other hand, is a centralized repository that allows you to store all your structured and unstructured data at any scale. It is usually a single store of data, including raw copies of source system data, sensor data, social data, etc, and transformed data used for reporting, visualization, advanced analytics, and machine learning. In particular, data lakes make it easy for data scientists to mine and interpret data, require minimal conversion, if any, to promote the detection of hidden patterns and business insights.
Organizations have embraced data lakes as the primary repository, as it eases the complexity of storing data in multiple formats. Data lakes look more relevant in healthcare due to the storage of different data formats such as patient records, lab results, Claims, Images, X-Rays, Clinical Notes, pharmacy data, etc.
Data is only useful if it can be used on time to help make decisions. For data-driven operations, a consumer or organization aiming to analyze data stored in a data lake will spend a lot of time locating it and preparing it for analytics. But it can be expensive to store data in a data warehouse, particularly if the data volume is high. On the other side, a data lake is planned for low-cost storage.
You’ll spend approximately 80% of the project time in just analyzing the data while designing machine learning models. Warehouses have built-in capabilities for transformation, making it simple and fast to perform this data planning, particularly on a big data scale. These warehouses can reuse features and functions across analytics initiatives, which means you can overlay a schema across various features. This decreases duplication and improves the quality of your results.
When the intention of data’s future usage is too uncertain or hypotheses are unpredictable and highly dependent on raw data, Data Lake aligns with organization’s need. Data warehouse is most suitable when organizations are pre-determined on the data usage and utilize structured data in daily business processes.
Want to know more about DL/DW mechanisms and the impact of big data analytics on your business? A chat with our data experts can be the ideal launch pad for your data driven endeavors.
Drop us a line at info@nalashaa.com.
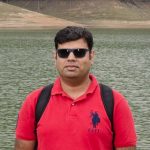
Pankaj Kundu
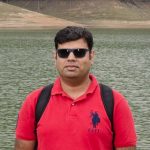
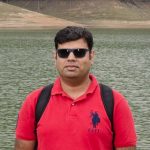
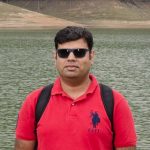
Latest posts by Pankaj Kundu (see all)
- Here’s How You Can Implement Transparency in Coverage - July 22, 2021